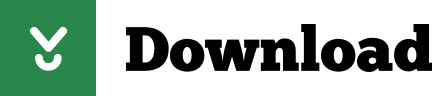
Since then it has progressed from the invention of dynamite to the use of heavy ANFO. Rock breakage with explosives has existed since the seventeenth century when black powder came into use in mining. The grade tonnage curve along with uncertainty will help mine management by improving understanding about the deposit. Ten different ore grade maps were generated for calculation of the uncertainty associated with the model. Therefore, lithological maps of the deposit were generated using sequential indicator simulation. The developed NN model incorporates lithological information as input parameters. The comparative result revealed that the NN model performed better for all four attributes of the case study mine than the ordinary kriging model. The performance of the model was tested and compared with an ordinary kriging model. The optimum hidden layer nodes were selected based on minimum mean square error of a validation data set. The learning parameters for the NN model were selected by genetic algorithm. A neural network (NN) model was used to develop a reliable estimate of ore grades of a study mine. A reliable estimate can represent the deposit close to reality. Ore grade evaluation is a decisive task in mine planning operations. The authors conclude by suggesting that the method described in this paper could be used for grade-control planning in ore deposits. These lithological maps were generated using indicator kriging. Prior to constructing the grade maps, lithological maps of the deposit at the unknown grid were prepared. After the superiority of the NN model had been established, it was used to predict the grades at an unknown grid location. This evaluation demonstrated that the NN model decisively outperformed the kriging model. To justify the use of the NN in the deposit, a comparative evaluation between the NN method and the ordinary kriging was performed. The input layer consisted of three spatial coordinates (x, y and z) and nine lithotypes, The output layer comprised all the grade attributes of limestone ore including silica (SiO 2), alumina (Al 2O 3), calcium oxide (CaO) and ferrous oxide (Fe 2O 3). The network was made up of three layers: an input, an output and a hidden layer. The NN model incorporated the spatial location as well as the lithological information for modeling of the ore body. This paper describes an attempt to improve the ore grade estimation through the use of a feed forward neural network (NN) model. The erratic nature of the deposit and the unavailability of adequate samples for each of the lithogical units made standard geostatistical methods of capturing the spatial variation of the deposit inadequate. Ore grade estimation for the limestone deposit was complicated by the complex lithological structure of the deposit. This study describes a method used to improve ore grade estimation in a limestone deposit in India.

MFS prediction equations based on PCA and SSE techniques were simple and suitable for practical use in overburden bench blasting of Indian coal mines.
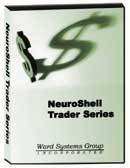
The results were tested and validated with the 19 actual blast data set at acceptable correlation levels and have been illustrated in the form of figures, tables and graphs.
#Neuroshell 2 crack mind trial
Hierarchical cluster analysis technique was used for confirming the similarity of blasting design parameters in two trial blasting data set. The PCA technique has shown good promise in eliminating the redundant parameters and in selecting relevant blast design parameters. The performances of these models were assessed through the determination coefficient (R²), F-ratio, standard error of estimate and root mean square error (RMSE). The two constructed and proposed models adequately selected relevant blast design, explosive and rock mass parameters. Mean fragment size (MFS) prediction models were subsequently developed using multiple linear regression (MLR) analysis technique. In the present study, principal component analysis (PCA) and stepwise selection and elimination (SSE) techniques were used to establish significant parameters (blasting design, rock and explosive) in surface coal mines by reducing dimensionality and variables from a host of blasting parameters.
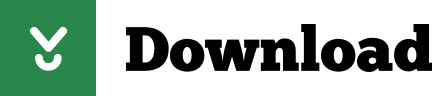